
Data Visualization with Power BI and R Visuals
A Look at JetBlue’s Most Profitable and Fuel Efficient Routes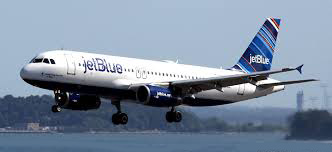
Data Visualization with Power BI and R Visuals has significantly stepped up the sophistication of data visualization. On July 5, 2016, the Power BI team announced support for R visuals in the Power BI Service. This is an update we’ve been anxiously awaiting!
Here’s a video we created that shows how you can create a data visualization with Power BI and R visuals to tell a story with data. In this example, we decided to look at the airline industry to determine the most profitable and fuel efficient routes. We analyzed the best and worst utilized domestic flights for all major carriers in 2015, followed by looking at monthly fuel costs. Air traffic statistics from the US Department of Transportation and Power BI helped us uncover the best and worst utilized airports by origin and destination.
Since the July 2016 update to the Power BI Service, you can now expand statistical data analysis with compelling visuals that integrate R. These new rich R visuals are fully integrated into Power BI service reports, and can be filtered, cross filtered, and pinned to dashboards.
Data Visualization with Power BI and R Visuals tells a story with data
The Utilization page is where we leveraged R to create a geography experience unlike any other BI tool. Using bar charts, we encoded Load Factor and Departures by color saturation for better detection of the best/worst utilized airports for the selected Airline (JetBlue). Selecting an airport from those charts cross-filters the R visual; a map rendered with the maps and geosphere R packages, sticking to the same color encoding for Load Factor and Departures, but adding different marks for origins and destinations. This is all accomplished with R, and successfully published to the Power BI service.
When we look at airlines with a high number of domestic flights, flight routes on the R visual can get very overcrowded. To aid with this, we added a field to the data model called Flight Occurrence, which not only helped make the geographic analysis more clear, but also more interesting. By introducing this new variable to the data model, we were able to do quick checks on Utilization between airports aren’t flown to very often, to airports that are flown to more than a hundred times a month.
Power BI analyzes factors for changes in fuel costs
The Fuel Cost page displays year-to-date KPIs at the top for JetBlue and a month-over-month graph which is actionable, plotting change in fuel costs along with additional tooltip information so airline personnel can address if fuel costs were a function of fuel price or distance traveled. Below the Month over Month graph, fuel costs are split by Aircraft to do a finer analysis on equipment being used and then further drill down to the flight level on the scatter plot. From our findings on the utilization page, we can do a search for those airports in question either by airport code or by airport name.
Power BI creates powerful visualizations out of the box, but now with R visuals, we can expand the visualization library to do a pixel perfect job on more sophisticated plots. By combining the interactive, user-friendliness of Power BI with advanced statistical data analysis produced in R, you can build great data analytics and share them with your team and to the world. Data Visualization with Power BI and R Visuals is the most exciting update to Power BI we’ve seen this year. Check out our Power BI and Data Visualization page to see more.
Do you want to tell a story with data? Please reach out to us and tell us more.
Our consultants are experts in BI, Big Data Analytics, Databases, and Cloud Solutions. Contact us in San Francisco to learn how you can harness your data.